We used eight baseline methods for comparison with our methodology, as follows:
Baselines:
- MCNN: a deep learning sleep staging method utilizing multivariate multimodal PSG signals.
- MMCNN: a multi-scale convolutional neural network for EEG signal classification.
- MLP+LSTM: a mixed neural network, which combines multilayer perceptron (MLP) and LSTM.
- DeepSleepNet: a mixed sleep staging method utilizing CNN and Bi-LSTM.
- TinySleepNet: an efficient and lightweight EEG sleep staging network.
- U-Time: a temporal fully convolutional network based on U-Net architecture for sleep staging.
- GraphSleepNet: a spatial-temporal graph convolutional neural network that can adaptively learn spatial-temporal features.
- Spatial-Temporal Transformer: a sleep staging method using transformer to extract spatial-temporal features.
Compared to other baseline methods, BSTT achieved the best performance on both datasets. Specifically, MCNN and MMCNN utilise CNN models to automatically extract sleep features, while RNN-based methods such as DeepSleepNet and
TinySleepNet focus on the temporal context in sleep data and model multi-level temporal features during sleep for sleep staging. In addition, GraphSleepNet and ST-Transformer simultaneously modelled the spatial-temporal relationships during sleep with
satisfactory results. However, GraphSleepNet and ST-Transformer cannot adequately reason about the spatial-temporal relationship, which limits the classification performance to a certain extent.
Our Bayesian ST-Transformer uses a multi-headed Bayesian relational inference component to reason about spatial-temporal relationships in order to better model spatial and temporal relationships. As a result, the proposed model achieves the best classification performance on different datasets.
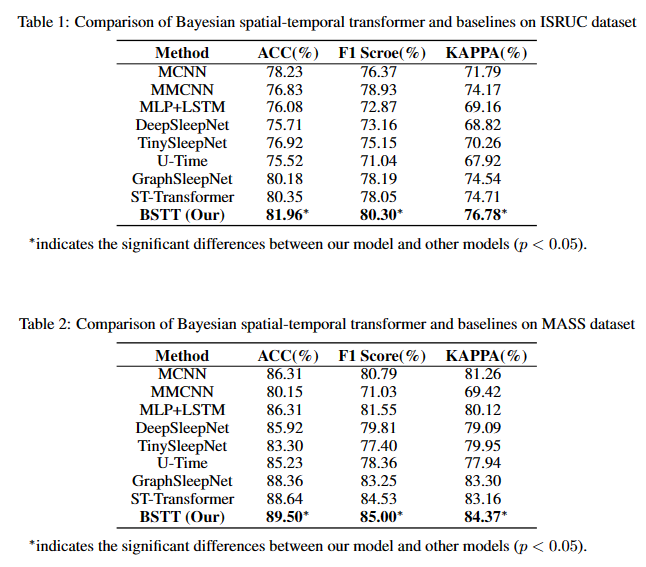 |
We visualize the spatial and temporal relationship graphs on the MASS-SS3 dataset. It has been revealed that during light sleep, cerebral blood flow (CBF) and cerebral metabolic rate (CMR) are only about
3% to 10% lower than
those of wakefulness while during deep sleep (Madsen & Vorstrup, 1991). Synaptic connection activity is
directly correlated with CBF and CMR, which is consistent with our spatial relationship intensity graphs. Besides,
Previous studies have shown that the stability of the unchanging period is stronger, and
sleep instability is the basis of sleep transition (Bassi et al., 2009), which is
consistent with our experimental results.